Introduction
One of the key attributes that defines a vehicle is its dynamic behaviour. Whether the main priority is providing the interaction that keen drivers crave or maximising ride comfort for those who simply want to get from A-to-B, the dynamic experience lies at the core of every vehicle’s DNA.
Fine tuning the systems and the fundamental design parameters that make up a vehicle’s ride and handling characteristics has always been an important task. It’s one that’s often dominated by subtleties that aren’t immediately apparent from raw data, instead requiring hands-on evaluation by experienced engineers and test drivers.
As the automotive industry evolves, this process is getting even more important. Incoming technologies, the move towards electrification and ever-increasing time and cost constraints are posing new challenges for OEMs and suppliers.
Driver-in-the-loop (DIL) simulation has proved itself to be an invaluable tool, both for confronting traditional challenges in the development process and for addressing these new opportunities. It enables a human driver to feel what the vehicle is doing – potentially long before a representative prototype exists in reality. This connection is vital if the chassis engineers are to drive the virtual model as they would on a real road or track. It not only provides a chance to gather subjective and objective feedback, but also guarantees authentic inputs into the vehicle model.
In this paper we shall discuss the benefits of using DIL simulation to test in the virtual world, the technology behind Ansible Motion’s state of the art modern simulators and how simulation can help the automotive industry adapt to a rapidly-changing world.
Feel the difference: Using DIL simulation to assess ride and handling
Download this white paper by completing this form.
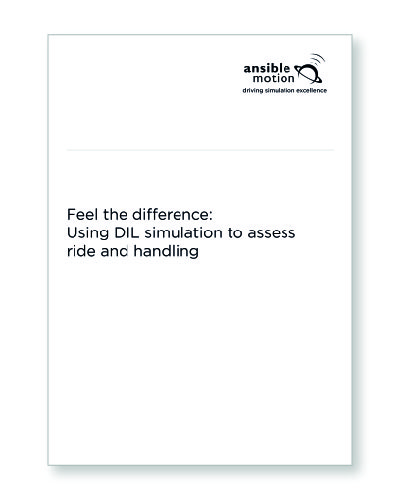
Get your copy
The best of both worlds
Traditionally, the automotive development process has relied on a mixture of real-world testing with prototype vehicles and laboratory-based rig testing. Testing in the virtual world with a DIL simulator combines the best of both worlds. It gives engineers the precise control of the boundary conditions offered by laboratory testing, along with the representative responses and wide range of different scenarios that come from placing a human driver in an open environment.
An obvious example is the weather. The simulation environment is able to model anything from an Arctic blizzard to a desert heatwave, with precise control and absolute repeatability. This doesn’t require a physical prototype to be shipped around the world and it’s not subject to unseasonable conditions or unpredictable weather changes.
Radically different car setups or weather conditions can be evaluated back-to-back within a matter of minutes. It’s also possible to tailor the test conditions to represent things that wouldn’t be possible in real life, such as an infinite loop that continually repeats the same sequence of corners or a world where it’s possible to disable temperature changes in the tyres.
This ability to fix or vary specific parameters as required by the test programme can be particularly useful when investigating potential conflicts. For instance, a setup change that improves the vehicle’s behaviour on warm, dry tarmac can immediately be checked on a cold, damp surface without leaving the room.
Fewer prototypes
The costs of physical prototyping begin long before the vehicles are shipped all over the world for testing. A single prototype vehicle can cost $250,000 (US) to $1m (US). With 50 to 70 prototypes built per development program, it’s estimated that automotive OEMs cumulatively spend $10bn (US) per year on prototypes.
Reducing the reliance on physical prototypes has a clear cost benefit for car manufacturers. What’s more, digital prototypes can be kept up to date with the latest design revisions as the project evolves. In contrast, physical prototypes have often tended to cascade down through various departments – with some groups getting access to the latest specifications whereas others may have to make do with earlier prototypes that are less representative of the finished product.
There is also a possibility that these highly valuable prototypes could be damaged or destroyed during testing. Worse still, there’s a risk that personnel could be harmed. Either could lead to significant setbacks in the development programme, particularly if there are only a limited number of physical prototypes available.
The importance of the driver
Many of the same observations about cost reduction, time efficiency and repeatability could be applied to offline computer simulation. But while this is a valid tool in some instances, there are other aspects that can only be appreciated by putting a human driver into the virtual world. The difference between a car being class-leading or merely average when it comes to a particular attribute can be extremely subtle. As a result, small but potentially crucial improvements may be hard to spot in raw data, whereas a skilled human driver will be able to detect these subtle nuances.
Part of this comes down to the complex relationship between the vehicle and the driver. As the vehicle’s behaviour changes, so too will the input from a human driver – for instance, turning in more aggressively if a new setup offers better response or more cautiously if the setup doesn’t inspire confidence. In contrast, an offline simulation with inputs based on a pre-determined open loop script or a basic model of driver behaviour, will not be able to adjust its responses in this characteristically human way.
It was this importance of capturing representative human behaviour that inspired Ansible Motion’s founder Kia Cammaerts to establish the company in 2009. Prior to that he had developed an offline simulation package for use in motorsport, which relied on a series of equations to predict how setup changes and design alterations would affect a vehicle’s lap time.
As time went on, Cammaerts took advantage of the increased computing power available to improve the detail in the equations. Beyond a certain point, however, adding more complexity into the equations no longer seemed to improve the accuracy of the prediction. His conclusion was that he had reached the point where the most significant factor was now the behaviour of the driver. It’s an observation that remains equally valid today, with the instinctive behaviour of a human driver still a vital part in obtaining representative results.
A clean sheet approach
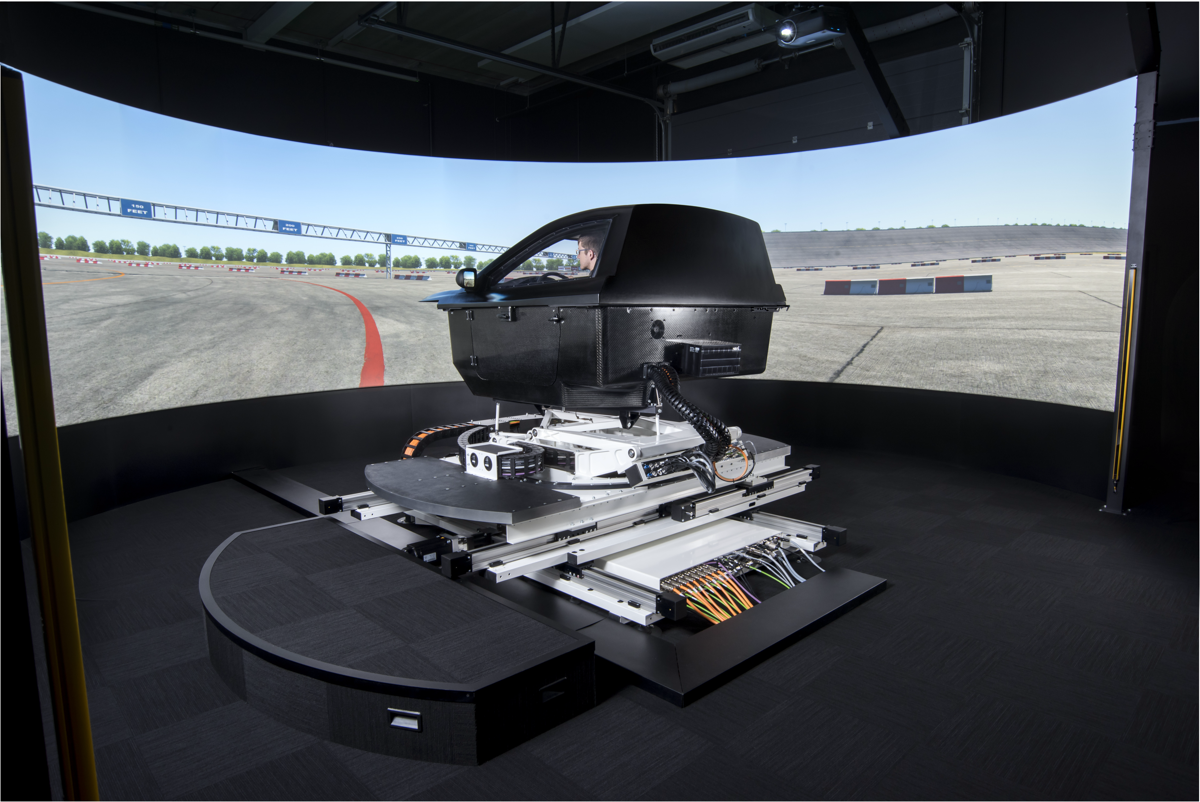
There’s a danger that any decisions taken at this early stage might prove difficult to reverse if the programme is based largely on physical prototypes. For instance, altering the hard points of the suspension can become extremely difficult once these have been laid down in the early prototypes – potentially forcing the development team to commit to a decision that they might later regret.
Using simulation provides engineers with a clean sheet, freeing them from these constraints. In one instance, an automotive OEM found that using Ansible Motion’s DIL simulators allowed it to bypass the first physical prototyping generation altogether. When the results were reviewed at the end of the programme the early digital prototype results were found to be more representative of the finished vehicle than would have been expected from a test mule at the same stage. This allowed more informed decisions to be made during the early stages of the project – the benefits of which were carried right through the development process.
Using virtual prototypes in the early stages not only removes the approximations that are sometimes involved with test mules, but also provides limitless freedom to experiment with different concepts. For instance, a manufacturer could evaluate the cost-to-benefit implications of adopting an entirely different suspension configuration or a new driveline layout on its next model.
This ability to approach projects with a clean sheet mentality is particularly useful as the car industry enters what promises to be a period of technological disruption, with new technologies such as battery electric powertrain prompting a fundamental rethink of vehicle design. Electric vehicles can have anything from one to four motors, driving one or more sets of wheels; the batteries might live under the floor of the cabin or down the backbone of the chassis; they might use mechanical differentials or software-driven torque vectoring. Even simple things like new packaging solutions influencing the driving position can have a big impact on the customer experience. It’s arguably the biggest shake up that the automotive industry has seen in a century and it opens up a whole new world of possibilities.
Another example is the arrival of intelligent chassis systems. Terrain-scanning active suspension, active anti-roll bars and rear-wheel steering are among the technologies that are becoming more commonplace as chassis systems evolve. Offline simulation of these features is limited to numerical predictions. In contrast, not only does DIL simulation provide more representative driver inputs for these quantitative studies, it also adds a much-needed qualitative impression.
It’s important to bear in mind that objective improvements won’t always result in a more pleasant or more involving experience for a human driver. For instance, there’s a risk that filtering out all imperfections from the road surface could leave keen drivers feeling detached from control of the vehicle or that a keener turn-in response could lead to the vehicle feeling nervous.
Looking further ahead, work is already taking place on advanced concepts such as high-level autonomy, alongside new mobility models like ridesharing. These will change the demands on vehicle chassis development, packaging and performance. The success of these concepts will depend heavily on the customer experience, which is a highly subjective topic, requiring an accurate and immersive representation of the vehicle to fully evaluate.
New challenges
A radical departure, such as producing the brand’s first all-electric vehicle, can be a step into the unknown for even the most experienced development teams. Without a comparable benchmark to reference, raw data alone can struggle to convey how a vehicle will behave. On the other hand, an experienced driver can step straight into an engineering-grade dynamic simulator and give meaningful feedback on a vehicle that is totally different to the company’s existing products.
When I got out of the simulator, everyone was waiting to hear what I was going to say. And I looked at the entire team and I said, ‘It’s not a Mustang’. And you could almost feel the air leave the room, with the gasps, and people thinking, ‘What did he just say?’
A good example of this came about in the development of the Ford Mustang Mach-E. This was a hugely important product for the brand, not just as its first all-electric vehicle, but one that would carry the iconic Mustang badge.
Dave Pericak, global director for icons at Ford, recalls the moment he first drove the car as a virtual prototype in the company’s Ansible Motion Delta Series DIL simulator.
I went back down [to the DIL simulator lab in North Carolina] and I re-drove what was now going to be the Mustang. It was awesome.
The team agreed that the fundamentals of the car were all in place, but Pericak’s verdict led to a complete rethink on the chassis tuning. When he returned he found a vehicle worthy of the Mustang name, he recalls.
Conclusion
There are some questions that can only really be answered by placing a human driver into the car. Doing so in a physical prototype can be expensive, it is reliant on the changeable conditions offered by the real world, and in the early stages of the project it can be quite a crude approximation to the finished product.
Digital prototyping with the use of DIL simulation has the potential to slash the time and cost required for the test programme; it frees engineers from the constraints of existing technology and gives them complete control over the test conditions, with absolute repeatability. As such, it often offers the best of both worlds, providing the controlled conditions of a laboratory test with the limitless variability of the real world.
Feel the difference: Using DIL simulation to assess ride and handling
Download this white paper by completing this form.
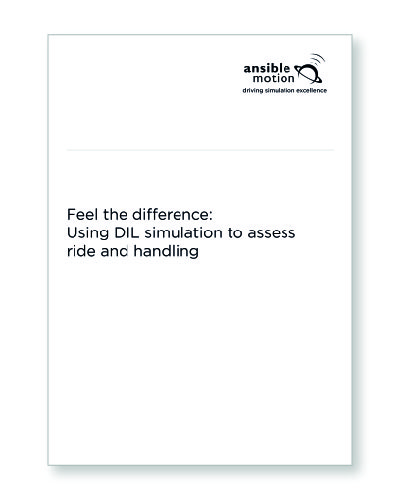